What’s That Termite? Artificial Intelligence Might Have the Answer
2021-09-30
興新聞張貼者
Unit秘書室
1,624
Source:2021-9-30/Entomology Today/Ed Ricciuti
In the not-too-distant future, a pest control technician prepares to deal with a termite infestation. His first order of business is to identify the species of termite, essential for effective management but not always an easy job nowadays because these little wood chewers are notoriously difficult to sort. For the tech of the future, however, it’s a snap. He pulls out his smart phone, clicks off a few pictures, and a souped-up version of machine learning fires back with an identification of the termite species at hand.
The scenario above is what a team of scientists in Taiwan hope will result from research, published in August in the Journal of Economic Entomology, that demonstrates how deep learning, in which a computer model identifies termite species, can be adapted for use via smartphone for pest control professionals and even homeowners. The system relies on what are called deep convolutional neural networks (DCNNs or CNNs) to extract and differentiate patterns within images and automatically identify termite species.
Says Jia-Hsin Huang, Ph.D., of the Institute of Information Science, Academia Sinica, in Taipei, Taiwan, “The termite-CNN model developed in the paper opens a window to introduce the effective machine-learning algorithms to termite pest control.”
The hardware and software comprising an artificial neural network is modeled after the way real neurons are arranged in the human brain. DCNNs mimic the processes in the cortex of the front lobe of the human brain that process angles, lines, and other features that combine to produce an image. Each artificial neuron handles a piece of the picture, so to speak, connecting with its counterparts to produce the full image captured by the eye. The algorithms used in deep learning systems can determine which features are associated with different species. The deep learning process depends on a classifier, an algorithm that the computer model uses to learn from data over which it has been trained, in this case for mobile applications. Once trained, the model can handle new data given to it.
A great number of images are required to train a model to distinguish different species. The Taiwan team employed smartphones to take 18,000 images of four termite pest species. Each image contained several termites so that, in the end, 24,000 individual images were used in development of the computer model. Senior author on the study Hou-Feng Li, Ph.D., of the National Chung Hsing University says, “The dataset is a group of photos, and each one [is] associated with a species name. In this study, we focused on the pest species in Taiwan, and hence we only used the commonly encountered four pests to train the model. It is easy to understand, for application, not many people need a software/model/app to identify all termite pests around the world.”
The termite researchers built their model on a framework, MobileNetV2, small enough to be used with smartphones. Describing the reasons for choosing that particular framework, Huang says, “MobileNet is designed for mobile devices. We could expect the future application of this deep learning model installed in the mobile phones to be used by the pest management officers and ordinary citizens in the real world. The architecture of MobileNet is developed by Google, and the model is open-source. Anyone could implement this model freely and train their own prediction models. Because the parameters of the deep learning models are huge and usually difficult to fine-tune with small batches of images, we have released our model parameters for future studies. As a result, any pest control professionals or laymen could use our trained parameters as initial values to train their own classification models of other pest species. It will reduce much efforts to train a new classification model and improve the performance in prediction.”
Deep learning is a natural for taxonomy and increasingly has been deployed to sort insects, but the research by the team from Taiwan is the first to do so with termites. Most classification of termites is based on their soldier or worker castes, as was done with this effort, because they are the castes most often encountered in the field. Even within a species, however, morphology and development of individuals can vary, influenced by factors such as age and environmental conditions. Reproductive adults, moreover, are often difficult to match with soldiers and workers. Termites from one geographic area, as well, may look different from their conspecifics elsewhere. There are, however, other clues to a termite’s identity than just its physical appearance. Behavior can be one. Two species used in the study, Coptotermes formosanus and Odontotermes formosanus, look alike, but soldiers of the former defend against ants while workers take on the foe for the latter.
There is no one-shot-cures-all for termite control, but it is not necessary to identify species. Control is tailored to the three different main groups of termites that are considered pests, determined by behavior and ecology: subterranean termites, drywood termites, and gardening and agriculture (or dampwood) termites.
To control subterranean termites in the urban environment, baiting and remedial treatment with liquid pesticide injections are the two most commonly used tools. However, current baiting tools are inadequate for controlling drywood termites. “However,” says Li, “to deliver the knowledge and options of control methods of a specific pest to a homeowner or farmer is still very difficult. The bottleneck is correct and efficient pest identification.”
Describing their objectives, the researchers write, “Our main contributions include the curation of numerous smartphone-based images of termite pests and the successful implementation of deep learning models for termite classification. Our ultimate goal is providing the termite image classifiers for installing in regular smartphones under commercial operation systems. We also have released the full model trained on our complete dataset so other researchers might benefit by the pre-trained parameters to develop sophisticated models including other termite species or different CNN architectures in mobile devices. The immediate identification result will lead the user to biological information of the termite pest, control options, control products, and even professional pest management service in reachable distance. The high identification efficiency and accuracy will improve the integrated pest management of termites.”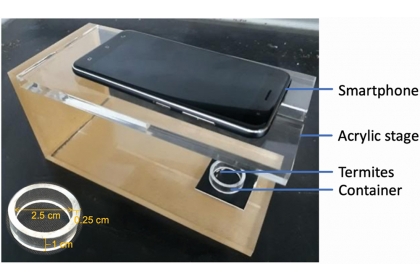
In the not-too-distant future, a pest control technician prepares to deal with a termite infestation. His first order of business is to identify the species of termite, essential for effective management but not always an easy job nowadays because these little wood chewers are notoriously difficult to sort. For the tech of the future, however, it’s a snap. He pulls out his smart phone, clicks off a few pictures, and a souped-up version of machine learning fires back with an identification of the termite species at hand.
The scenario above is what a team of scientists in Taiwan hope will result from research, published in August in the Journal of Economic Entomology, that demonstrates how deep learning, in which a computer model identifies termite species, can be adapted for use via smartphone for pest control professionals and even homeowners. The system relies on what are called deep convolutional neural networks (DCNNs or CNNs) to extract and differentiate patterns within images and automatically identify termite species.
Says Jia-Hsin Huang, Ph.D., of the Institute of Information Science, Academia Sinica, in Taipei, Taiwan, “The termite-CNN model developed in the paper opens a window to introduce the effective machine-learning algorithms to termite pest control.”
The hardware and software comprising an artificial neural network is modeled after the way real neurons are arranged in the human brain. DCNNs mimic the processes in the cortex of the front lobe of the human brain that process angles, lines, and other features that combine to produce an image. Each artificial neuron handles a piece of the picture, so to speak, connecting with its counterparts to produce the full image captured by the eye. The algorithms used in deep learning systems can determine which features are associated with different species. The deep learning process depends on a classifier, an algorithm that the computer model uses to learn from data over which it has been trained, in this case for mobile applications. Once trained, the model can handle new data given to it.
A great number of images are required to train a model to distinguish different species. The Taiwan team employed smartphones to take 18,000 images of four termite pest species. Each image contained several termites so that, in the end, 24,000 individual images were used in development of the computer model. Senior author on the study Hou-Feng Li, Ph.D., of the National Chung Hsing University says, “The dataset is a group of photos, and each one [is] associated with a species name. In this study, we focused on the pest species in Taiwan, and hence we only used the commonly encountered four pests to train the model. It is easy to understand, for application, not many people need a software/model/app to identify all termite pests around the world.”
The termite researchers built their model on a framework, MobileNetV2, small enough to be used with smartphones. Describing the reasons for choosing that particular framework, Huang says, “MobileNet is designed for mobile devices. We could expect the future application of this deep learning model installed in the mobile phones to be used by the pest management officers and ordinary citizens in the real world. The architecture of MobileNet is developed by Google, and the model is open-source. Anyone could implement this model freely and train their own prediction models. Because the parameters of the deep learning models are huge and usually difficult to fine-tune with small batches of images, we have released our model parameters for future studies. As a result, any pest control professionals or laymen could use our trained parameters as initial values to train their own classification models of other pest species. It will reduce much efforts to train a new classification model and improve the performance in prediction.”
Deep learning is a natural for taxonomy and increasingly has been deployed to sort insects, but the research by the team from Taiwan is the first to do so with termites. Most classification of termites is based on their soldier or worker castes, as was done with this effort, because they are the castes most often encountered in the field. Even within a species, however, morphology and development of individuals can vary, influenced by factors such as age and environmental conditions. Reproductive adults, moreover, are often difficult to match with soldiers and workers. Termites from one geographic area, as well, may look different from their conspecifics elsewhere. There are, however, other clues to a termite’s identity than just its physical appearance. Behavior can be one. Two species used in the study, Coptotermes formosanus and Odontotermes formosanus, look alike, but soldiers of the former defend against ants while workers take on the foe for the latter.
There is no one-shot-cures-all for termite control, but it is not necessary to identify species. Control is tailored to the three different main groups of termites that are considered pests, determined by behavior and ecology: subterranean termites, drywood termites, and gardening and agriculture (or dampwood) termites.
To control subterranean termites in the urban environment, baiting and remedial treatment with liquid pesticide injections are the two most commonly used tools. However, current baiting tools are inadequate for controlling drywood termites. “However,” says Li, “to deliver the knowledge and options of control methods of a specific pest to a homeowner or farmer is still very difficult. The bottleneck is correct and efficient pest identification.”
Describing their objectives, the researchers write, “Our main contributions include the curation of numerous smartphone-based images of termite pests and the successful implementation of deep learning models for termite classification. Our ultimate goal is providing the termite image classifiers for installing in regular smartphones under commercial operation systems. We also have released the full model trained on our complete dataset so other researchers might benefit by the pre-trained parameters to develop sophisticated models including other termite species or different CNN architectures in mobile devices. The immediate identification result will lead the user to biological information of the termite pest, control options, control products, and even professional pest management service in reachable distance. The high identification efficiency and accuracy will improve the integrated pest management of termites.”
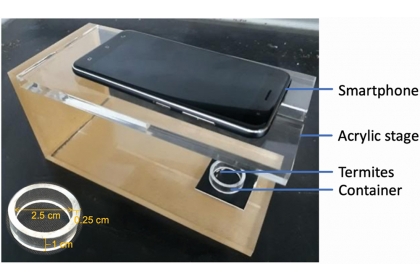